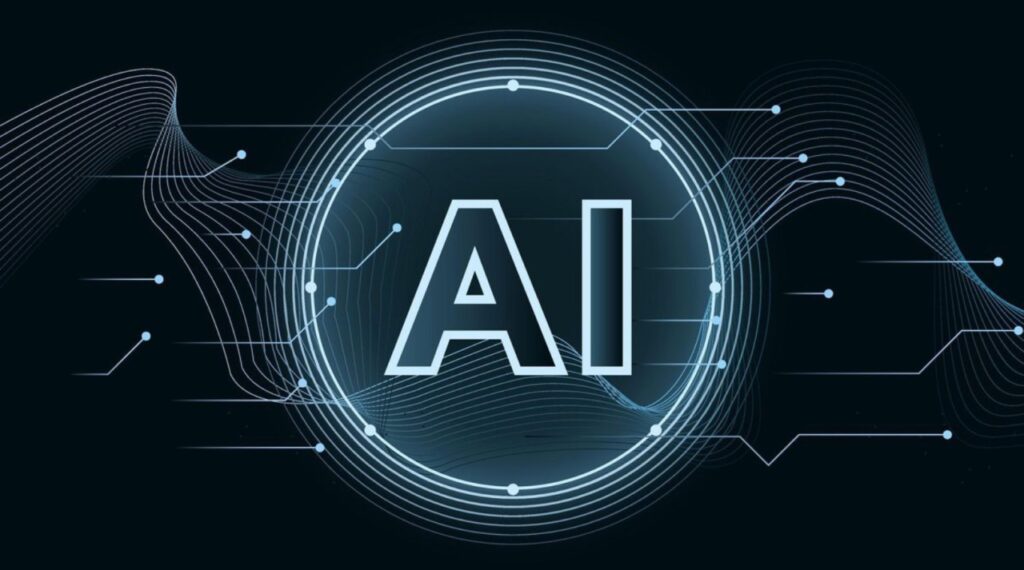
Network Detection and Response (NDR) has emerged as a critical component in modern cybersecurity frameworks, providing visibility into network traffic to identify and respond to threats. With cyber-attacks becoming more sophisticated, traditional security measures are falling short.
Threat actors are taking the form of elaborate, multi-stage attacks that can bypass traditional methods of detection. The changing threat landscape and the growing sophistication of cyber-attacks has made it imperative for organizations to move away from legacy network security, leading to interest in Artificial Intelligence (AI) and Machine Learning (ML) technologies that have redefined NDR solutions from reactive prevention solutions to proactive defense players to detect unknown threats before they can inflict damage.
How AI and Machine Learning Enhance NDR
- Behavior analysis – ML algorithms don’t just look for known threats; they learn what’s “normal” for your network and flag unusual activity. This means catching those hidden attacks that signature-based systems completely miss.
- Fast processing – AI-powered NDR handles massive traffic volumes in real-time, so nothing slips through the cracks while your system is “thinking.” This makes processing faster and more accurate.
- Getting ahead of attacks – Instead of just reacting after you’ve been attacked, ML models actually predict where threat actor might be lurking based on subtle patterns they’ve learned from past attacks. It’s like having a security team that can see around corners.
- No more alert fatigue – Traditional systems bombard teams with false alarms and that is becoming a big problem for SOCs. AI with ML is remarkably good at filtering out the noise so your team can focus on genuine threats instead of chasing ghosts all day.
Key Benefits of AI-Driven NDR
- Higher Efficiency: Automation handles the tedious, repetitive analysis tasks that used to consume entire workdays. We’ve seen teams cut security tickets by nearly half and reclaim thousands of staff hours annually.
- Scalability: ML-powered NDR scales with growing networks, adapting to new devices and traffic without requiring constant manual updates and rule-writing.
- Global threat intelligence: Your NDR solution isn’t fighting alone. It’s constantly learning from attacks happening worldwide, so when a new threat emerges in Singapore, your system in Chicago is already prepared for it.
- Precision targeting: ML excels at distinguishing between harmless anomalies and genuine threats, dramatically reducing those frustrating false positives that plague traditional systems.
- Automated Response Capabilities: Modern NDR doesn’t just detect threats – it automatically contains them. Whether isolating compromised devices or blocking command-and-control traffic, your defense system works 24/7, even when your team is offline.
Challenges and Considerations
While the benefits of incorporating AI and Machine Learning in your Network Detection and Response (NDR) are formidable, there also are challenges in practical deployment. Here are several specific challenges and considerations that organizations should carefully consider:
- Implementation Complexity: Artificial Intelligence and its management require skill and expertise It isn’t a matter of just plugging in a system — businesses require skilled professionals who know how to set it up properly and ensure that it matches with infrastructure that is existing.
- Danger of Over-Reliance: AI is a terrific tool, but not a perfect one. Relying too much on it without human checks could create chinks — a bit like putting too much faith in autopilot with the pilot ready to take the wheel.
- Adversarial Attacks: Cybercriminals are clever, and some are already working to outsmart AI with tactics designed to confuse its models. It’s a cat-and-mouse game that demands constant vigilance.
- Resource Demands: Running AI-driven analysis in real time isn’t light work—it requires serious computing power. For some organizations, keeping up with those demands could strain budgets and systems alike.
Future of AI & ML in NDR
The evolution of NDR technology continues at a rapid pace. Self-learning algorithms increasingly adapt to network environments without human intervention, continuously improving detection accuracy while reducing management overhead. These advances enable NDR to detect subtle attack indicators that would otherwise remain hidden.
Integration with complementary security technologies represents the next frontier. NDR solution now converge with zero trust architectures and extended detection and response (XDR) platforms, creating unified security ecosystems that eliminate visibility gaps between security domains.
As threats evolve, so will AI-driven NDR. The ongoing arms race between attackers and defenders drives continuous innovation, with ML models becoming increasingly sophisticated at identifying attack patterns while resisting adversarial techniques designed to confuse AI systems.
Conclusion
NDR has also evolved from being a simple monitoring tool to a way to detect and respond to threats, driven by trends in AI and ML. For resource-constrained organizations struggling to keep pace with increasingly sophisticated attacks, AI-powered NDR enables the real-time visibility and automation required to maintain a strong security posture. As cyber threats continue their evolution, AI-driven network security has become not merely an advantage but a necessity for organizations serious about protecting their digital assets.
Solutions like Fidelis Network® stand at the forefront of this transformation, offering advanced threat detection, complete network visibility, and automated response capabilities that enable organizations to detect, hunt, and respond to threats with unprecedented speed and accuracy.